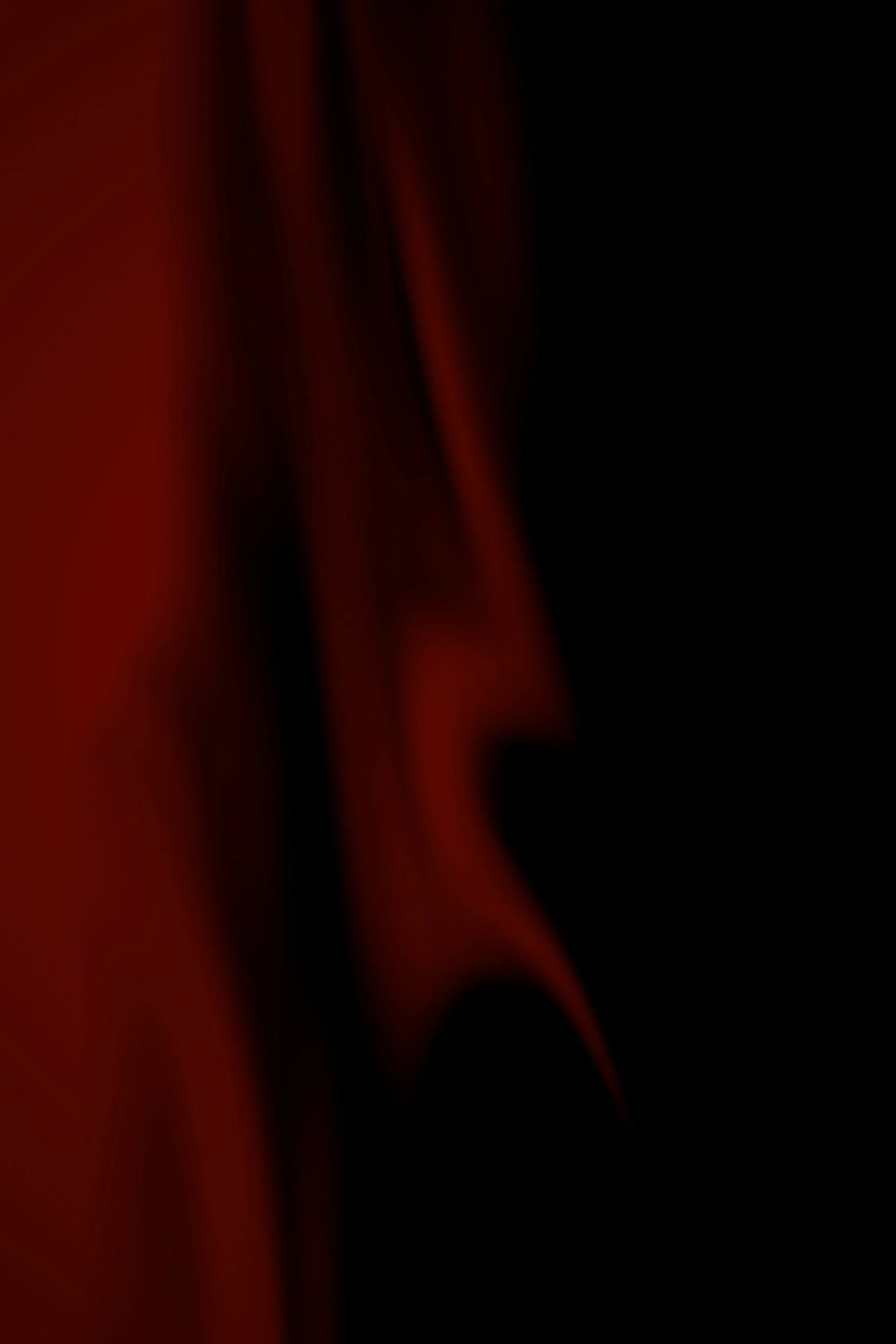
How Likely are Attacks on AI?
Led by Mileva Security Labs, conducted in partnership with the Australian National University (ANU) and the University of New South Wales (UNSW) and generously funded by the Foresight Institute.
AI systems are vulnerable to both cyber and new AI-specific attacks. But how often are AI systems attacked? How often are attacks successful? And how should we update our risk management processes to address this emerging AI cyber risk?
This project analyses media reports of AI incidents ‘in the wild’ to investigate the likelihood of AI Security threats.
Executive Summary
This research aims to quantify the likelihood of AI security incidents in real-world industry settings. This work is driven by a gap in AI risk management; risk is traditionally calculated as the product of severity and likelihood, yet the likelihood of AI security incidents remains poorly understood.
This work feeds into broader initiatives to ensure the safe and responsible future of AI technologies. AI security focuses on those incidents where an external threat actor (human or AI) seeks to disrupt, deceive or disclose information from AI systems and models. Criminals, nation state actors and hackers target AI models to evade detection (such as malware and spam filters), to force a specific classification (fraudulently bypassing AI-driven biometric authentication) or to leak sensitive information from training data or model parameters. Typically seen as lying at the intersection of cyber security and responsible AI efforts, this threat is taken extremely seriously, as evidenced by the United States investment in an AI Security Centre and MITRE’s new AI Incident Sharing Initiative. We cannot have safe AI unless we have secure AI.
The likelihood of AI security incidents is underexplored as the majority of existing research into AI security attacks occurs in experimental settings. Efforts to adapt existing cybersecurity frameworks to AI security have been constrained by two key challenges: a lack of historical data on the frequency of AI security incidents and a lack of evidence-based mapping of AI-specific vulnerabilities against the factors related to likelihood. Risk management practice is essential to maturing the AI industry - while Government agencies and researchers are aware of the threat, investment in such initiatives by private companies is driven by risk, and without sufficient risk management best practice, those companies that are the main developers and users of AI may not be sufficiently incentivised to ensure their security.
This project emphases real-world applicability by drawing insights from incidents ‘in the wild’ and centering practical, best-practice approaches to likelihood from various fields. This interim report outlines our findings to date in analysing 69 publicly disclosed AI security incidents and assessing the applicability of cybersecurity, insurance, and compliance likelihood practices.
Methodology
The following approach was used to investigate the relationship between likelihood and AI Security.
AI Incident Database
Narrative literature review of AI security and risk management literature to understand the current state of AI security likelihood related research.
Systematic analysis of AI security incidents, compiled from various sources and enriched against key drivers.
Mapping incidents to established risk frameworks such as the Common Vulnerability Scoring System (CVSS).
Summary statistics and exploratory data analysis of the incidents.
Development of hypotheses to inform the next steps of the project.
AI Incident Risk Analysis
A structured survey of 112 employees of organisations using AI, enriched with 25 interviews to understand their current AI security awareness and practices.
Application of five different risk modelling techniques to the incident database (risk matrices, Bayesian methods, Laplace, Monte Carlo and Machine Learning (decision tree)) - not included in this report but these initial results will be shared offline as part of the consultation process.
Initial Results
1. Inconsistency in Defining AI Security Incidents
Our study found strong evidence through both our literature review and analysis that there is a lack of consistent taxonomy, encompassing diverse cases such as cyberattacks on AI systems, attacks on ML models, and malicious uses of AI. This conflation of AI system security with model-specific vulnerabilities highlights the need for a robust framework.
2. Under-Reporting of AI Security Incidents
Our literature review and initial results led us to develop the hypothesis that true AI security incidents may be under-reported due to their complexity and lack of categorisation. The absence of a centralised repository for AI-specific threats, combined with the difficulty in identifying subtle disruptions, suggests that many incidents go unnoticed. This line of research is something we intend to develop further.
3. Bespoke Models may be at Greater Risk (or least targeted in different ways to third party AI)
Preliminary findings indicate bespoke models may be more vulnerable than third-party AI systems to certain attacks. Incidents involving poisoned datasets or malicious executables in bespoke models were particularly impactful. However, further research is needed to validate these observations.
4. Challenges in AI Risk Modelling
Current cyber risk models are limited in their application to AI. They rely on assumptions and lack validation mechanisms, and the existing best practice (risk matrices) may be too simplistic. The distinction between hazards and events in AI security remains a critical area for further exploration.
5. Lessons from Emerging Technologies
The evolution of technologies such as the internet, IoT, and cryptocurrency highlights parallels in risk management. Game theory offers a promising approach to understanding the strategic behaviour of attackers, particularly given the rapid and widespread adoption of AI without mature risk mitigations.
6. Utility of Likelihood in Risk Assessment
Likelihood analysis, crucial for risk calculations, is limited by sparse AI-specific data and the unique vulnerabilities of AI systems. Adapting cybersecurity methodologies and improving data availability are necessary steps to make likelihood assessment more actionable.
Impact
This research is already contributing to the broader AI security field:
Collaboration with organisations developing AI security standards: Input from MITRE ATLAS and OWASP teams has informed the approach and validated initial findings.
Dissemination among key AI security stakeholders: Findings will be presented to the AI security community at the UK’s National Cyber Security Centre (NCSC) AI Security Conference.
Academic Contributions: Two papers are being prepared for submission to the International Conference on Machine Learning (ICML).
Next Steps
This research elucidated many initial insights that we intend to pursue until the end of the funding period, and ideally beyond that period as well.
First, we are communicating our initial findings to as many researchers, practitioners and policy-makers as possible. Our next steps will be guided by what the community will find most useful, collaboration with other organisations (particularly where we might gain access to additional data), and aligning our approach with best practice. To this end, we have already sought feedback from the National Cyber Security Centre (NCSC), MITRE ATLAS, OWASP and a number of other organisations. We are presenting this work at the WAIST conference in Manchester, Society of Risk Analysis Conference in Melbourne, Australia, and are submitting papers based on this research to ICML and Black Hat USA.
Second, we intend to further enrich the data by conducting structured interviews with researchers that discovered the events listed, and with those organisations impacted. We already conducted a survey and interview of 112 employees of organisations using AI and found only 30% of technical respondents could confirm if their organisation considers AI security. We hope to extend this research further through the paradigms of understanding these incidents further.
Third, we have already applied several risk modelling approaches and compared their efficacy, including traditional risk matrices, Bayesian models, Monte Carlo models, and machine learning approaches. These results are not included in the report however we will be seeking feedback on these results through the feedback process, and this analysis will be completed by the end of the project period (July 2025).
Beyond July 2025 we see several areas of significance that could further the impact of this work. In particular we see significant potential in:
Surveys, interviews, and media analysis to uncover additional AI security incidents that may not be reported in existing databases,
Game theory approaches to understand which systems and models adversaries are more likely to target,
Threat intelligence and OSINT research (including dark web analysis) to understand what AI targets and tools are being discussed or offered among adversarial networks,
Integrating and aligning our initial risk modelling approaches to other fields (insurance, cyber, finance) to further refine them,
Closer integration of these risk modelling approaches with AI safety fields like evals to both learn from and contribute to efforts in the safety and alignment space.
Seeking Input
This interim report represents an evolving body of work. We are actively seeking input from industry, academia, and policy stakeholders to refine our findings and create a comprehensive final report. Contributions will help shape future recommendations and frameworks for AI security.
We welcome responses to these questions (and any others):
Do you have access to additional information or datasets we could use to shape this analysis?
Can you put us in touch with other researchers or practitioners informing AI risk management best practice?
Let’s talk ocllaboration to integrate this research with other works pursuing similar goals in AI safety and cyber security?
Project lead: Harriet Farlow
Harriet Farlow is the founder of Mileva Security Labs and her PhD is in adversarial machine learning. She has worked at the intersection of AI and security for a decade, in consulting, tech start-ups, and the Australia Government. Harriet’s work bridges technical research and policy, and she aims to empower organisations to navigate the complex landscape of AI security. She has spoken at DEF CON and other leading forums, advocating for practical and scalable AI risk solutions.
Researcher: Tania Sadhani
Tania Sadhani is an AI security researcher at Mileva Security Labs and an Honours student in Machine Learning at ANU. With a strong focus on adversarial threats and AI misuse, Tania contributes to cutting-edge research on AI risk and security frameworks. She is passionate about advancing methodologies that ensure AI systems are both safe and resilient.